ML & AI
Flexible Pricing Plans
Real-Time Forecasting
- Real-Time Data Integration
- Dynamic Prediction Models
- Applications in Various Domains (Retail, Finance, Healthcare, Logistics, etc)
- Event-Driven Forecasting
- High-Performance Computing
- Visualization and Dashboards
- Real-Time Anomaly Detection
- AI and IoT Integration
- Industry-Specific Solutions (E-commerce,Energy, Transportation)
Sales Pipeline Forecasting
- Initial Consultation and Objective Definition
- Data Collection and Integration
- Define Sales Pipeline Stages
- Analyze Historical Data and Conversion Rates
- Estimate Future Sales Opportunities
- Apply Probabilities to Opportunities
- Scenario Analysis
- Forecast Revenue and Sales Volume
- Review and Adjustment with Sales Team
- Continuous Monitoring and Refinement
Scenario-Based Forecasting
- Initial Consultation and Objective
- Data Collection and Preprocessing
- Feature Engineering
- Model Selection and Training
- Scenario Definition and Simulation Setup
- Forecast Generation and Comparison
- Sensitivity Analysis
- Decision Support and Risk Assessment
- Visualization and Reporting
- Continuous Monitoring and Model Adjustment
We Provide Best
Machine Learning and Artificial Intelligence
Predictive Modeling
Classification and Clustering
Recommendation Systems
Anomaly Detection
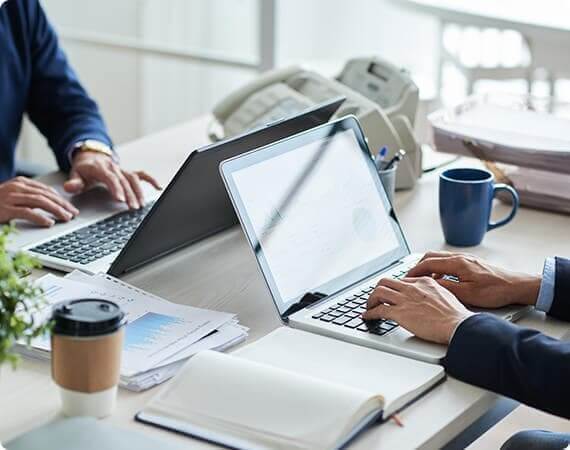
We Organize Our
Production Process
Analysis in machine learning and AI involves training models using historical data and evaluating their accuracy and performance. Data scientists analyze patterns and behaviors to build models that can make predictions or classify information. Advanced techniques like feature engineering, hyperparameter tuning, and model evaluation metrics ensure that the AI systems are accurate and aligned with real-world scenarios.
The design phase in machine learning and AI focuses on developing models and systems that align with business goals. This includes selecting the right algorithms, designing the architecture, and structuring data pipelines. A well-designed machine learning or AI system ensures efficient data processing, optimal model performance, and seamless integration with existing platforms.
Testing in machine learning and AI ensures that models function as expected and generalize well to new, unseen data. This includes cross-validation, performance testing, and checking for model biases. Rigorous testing helps identify issues like overfitting or underfitting, ensuring the model is reliable, scalable, and capable of delivering actionable insights in production environments.
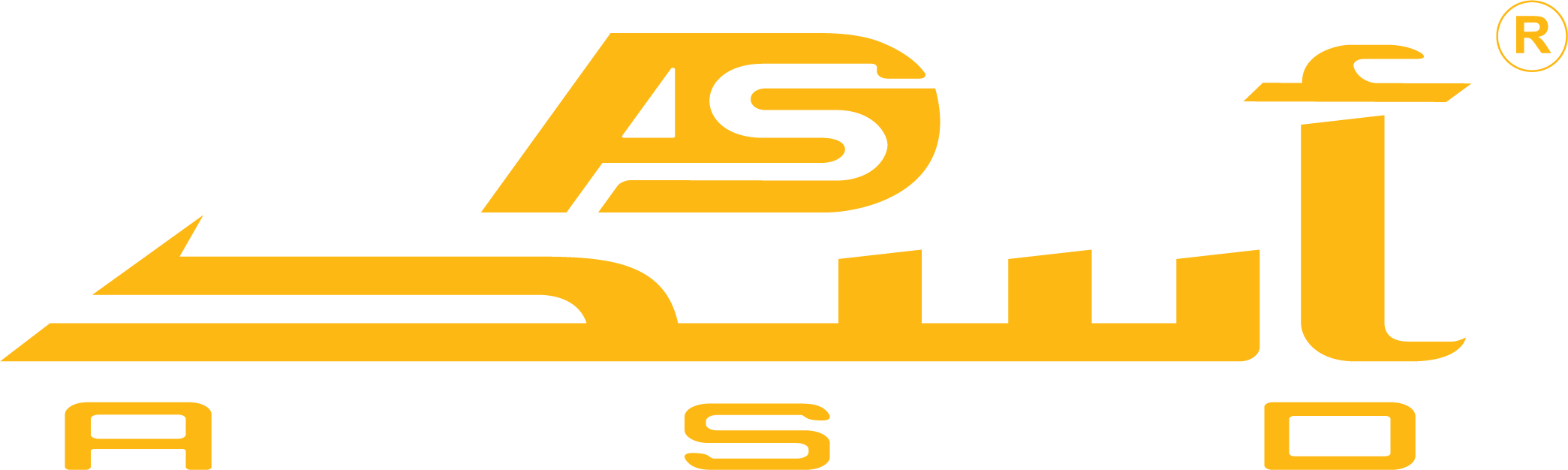
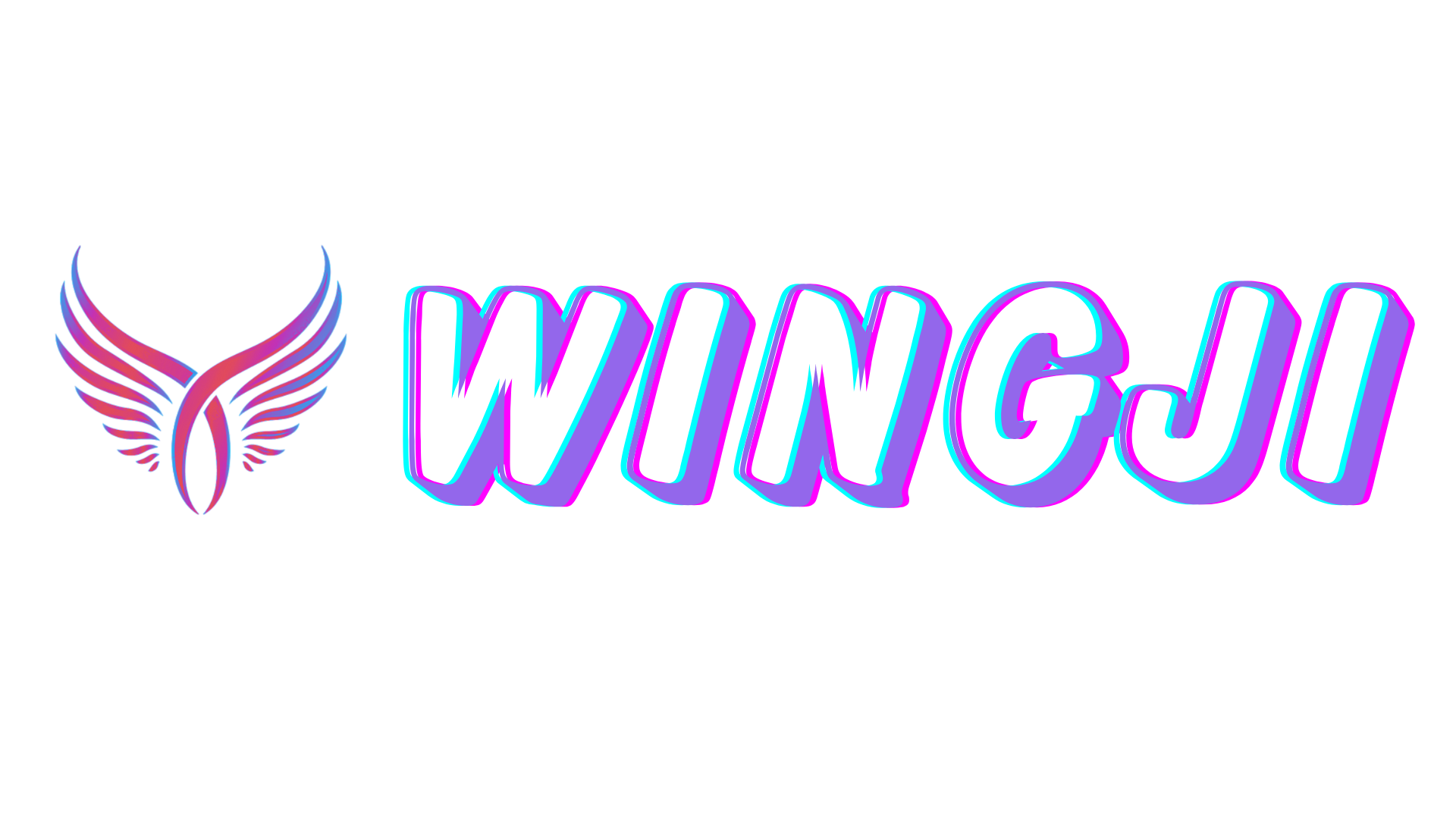
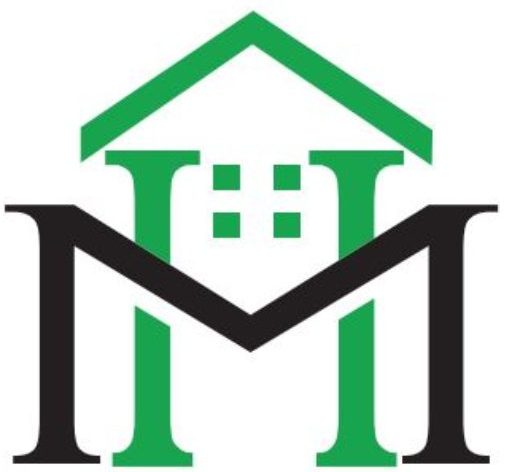
